Why AI Detectors Don't Work: Unveiling the Limitations
Educators, publishers, and other professionals are turning to AI detectors in hopes of maintaining integrity, but the effectiveness of these tools has come into question.
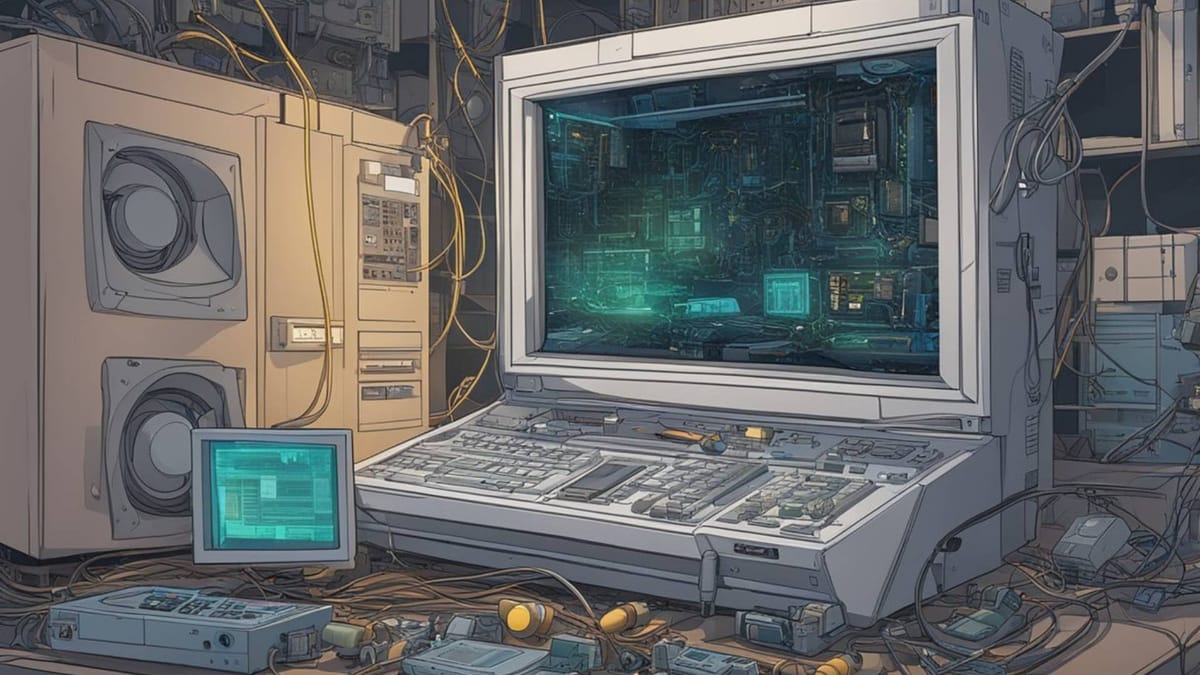
As AI becomes more prevalent in various sectors, the question of authenticity and originality in written work has risen to the fore. Educators, publishers, and other professionals are turning to AI detectors in hopes of maintaining integrity, but the effectiveness of these tools has come into question.
AI detectors such as have surfaced as a proposed solution to identify content created by machines. However, the task of detection is not as straightforward as it might seem. Numerous reports, including an article from MIT Sloan, highlight that AI-written text is becoming increasingly indistinguishable from that of human authors. This similarity makes the job of AI detectors exceptionally challenging, leading to a significant amount of both false positives and false negatives.
The reality faced by individuals relying on these detectors is one of uncertainty, as the tools may provide misleading outcomes. A piece from Ars Technica confirms the low accuracy rates, undermining the confidence in AI detectors. This situation calls for a closer examination of the underlying technologies of these tools and a discussion on alternative approaches to ensuring the authenticity of text in the age of AI.
Fundamentals of AI Detection
AI detectors are designed to differentiate between content generated by artificial intelligence and that which is authored by humans.
What are AI Detectors
AI detectors refer to tools or systems that employ algorithms to distinguish AI-generated text from human-written content. These detectors analyze patterns, consistency, and linguistic nuances that are characteristic of AI output, which can often lack the idiosyncrasies of human language. The ultimate aim of an AI detector is to flag content that appears to be generated by an AI, often providing a confidence score to indicate the likelihood of AI authorship.
AI Detector tools:
Here is a list of tools you can use to paste your content in to check if it comes up as human or AI:
Expectations vs. Reality
The expectations surrounding AI detectors commonly assume a high degree of accuracy in identifying AI-generated text. However, the reality is that these tools face substantial challenges, with some studies showing detection rates as low as 26% for correctly identifying AI-written content. Several factors contribute to this, including the increasing sophistication of AI writing tools and inherent limitations in the detectors' algorithms. Furthermore, the interpretation of analysis results is nuanced: a confidence score reflects a probability, not a certainty, adding layers of complexity to the discernment process.
Challenges in AI Detection
Detecting AI-generated content is fraught with various challenges, from the intricate nature of AI's creation process to the evolution and adaptation of technologies aimed at eluding detection. Here are some specific hurdles:
Complexity of AI Algorithms
AI algorithms are designed to mimic human writing, which inherently makes them intricate and capable of producing varied and sophisticated outputs. Techniques like deep learning have enabled AI to learn from vast amounts of data, making the task of distinguishing AI-generated text from human-generated text exceptionally difficult. Machine learning models, such as GPT-3, are trained on diverse internet text, so they can effectively generate content that appears naturally written, often indistinguishable from a human's craft.
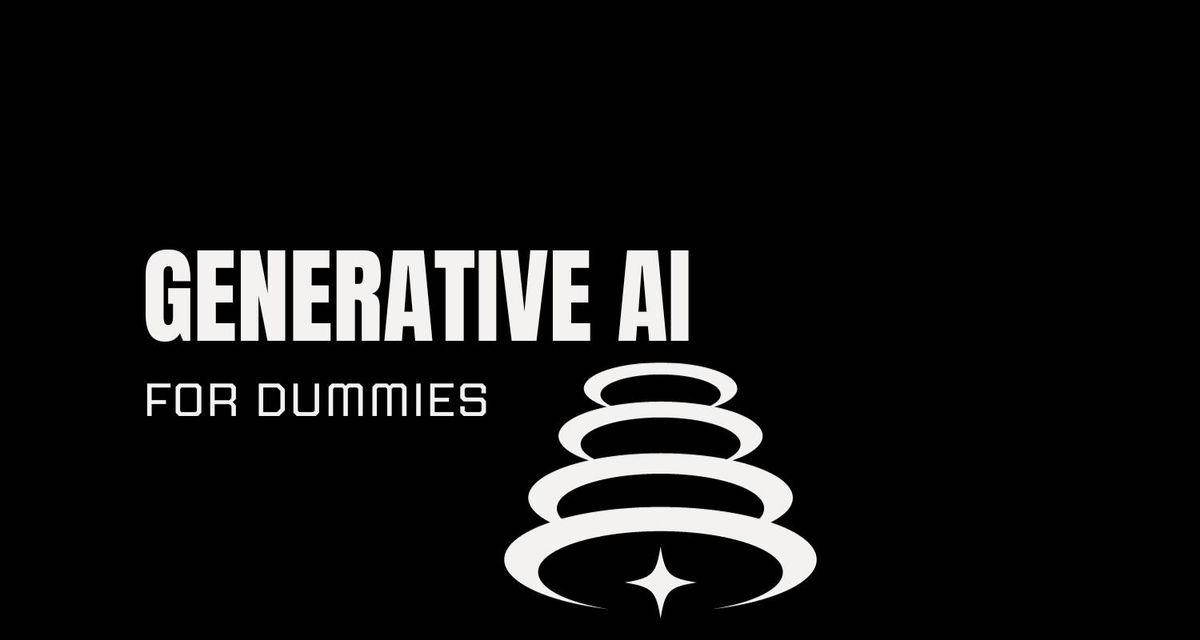
Adversarial Attacks
AI systems can also be subjected to adversarial attacks, where they are intentionally fed with misinformation or confounding patterns that lead to incorrect results. Adversaries may purposefully insert subtle cues or modify text in ways that make it harder for detection models to spot AI-generated content despite efforts to create robust detectors. These modes of deception continually evolve, staying one step ahead of detection technologies.
Data Quality and Availability
The effectiveness of AI detectors is heavily dependent on the quality and availability of data they are trained on. Datasets with comprehensive and representative samples of AI and human text are vital for training robust detectors. However, access to such datasets can be limited due to privacy concerns and proprietary restrictions. Additionally, AI models rapidly evolve, which can make existing datasets obsolete, thus continually challenging the accuracy and reliability of AI detectors.
These factors contribute to the innumerable challenges faced in the perpetual cat-and-mouse game of AI detection, necessitating ongoing vigilance and innovation in counter-detection measures.
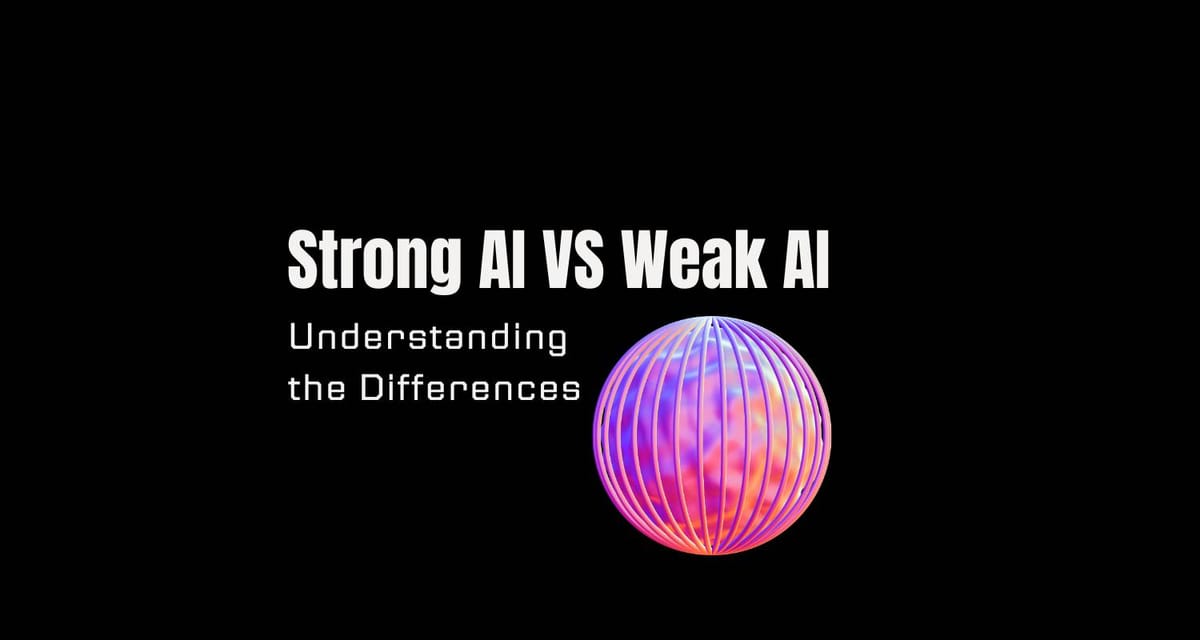
Software Reliability
Software reliability plays a critical role in the operational effectiveness of AI detectors. Software designed to identify AI-generated text faces several hurdles:
- Algorithmic Complexity: The sophisticated patterns of AI-generated text require complex algorithms that can be prone to errors or omissions.
- Adaptability: As AI content generation evolves, detection software may struggle to keep pace with newer methodologies.
Each factor plays a part in the ongoing challenges that AI detectors face today.
Ethical and Privacy Concerns
When it comes to AI detectors, two significant areas of concern are how the data is handled ethically and how privacy is maintained. Users may not be fully aware of how their data is used, and the technology could inadvertently reinforce existing biases.
User Consent Issues
Typically, AI detectors require access to vast amounts of text to determine if content is AI-generated. User consent becomes a pivotal issue, as individuals might not have agreed to have their writings scrutinized or used for model training. For example, AI detectors developed by academic institutions might use essays or papers submitted by students without explicit permission, encroaching on their intellectual property rights.
Bias and Discrimination
AI detectors can inadvertently embed bias within their results. This bias often reflects historical data inequalities or algorithmic discrepancies. For instance, outputs from non-native English speakers may be more prone to being classified as AI-generated due to linguistic idiosyncrasies. Moreover, writing styles that deviate from the 'norm' used to train these detectors can be unfairly flagged, leading to potential discrimination against certain groups of writers.
Future Prospects
In the pursuit of enhancing AI writing detectors, researchers and policymakers are considering innovative approaches and frameworks.
Improving Detection Methods
Researchers are working on advancing algorithms and using large datasets to train AI to distinguish humans from AI-generated text better. For example, OpenAI acknowledges that its detector's efficacy is a work in progress and that there's significant room for improvement. Artificial intelligence's ability to understand context and subtleties in language could be pivotal in improving detection methods, particularly as AI-generated writing becomes more sophisticated.
Legislation and Regulation
The emergence of more robust AI writing detectors may soon be supported by legislative efforts aiming to standardize practices.
For instance, regulators might impose requirements for AI to self-identify, creating a legal framework to discourage deception. Moreover, there's an evolving discussion around the ethical use of AI, which suggests that future regulation could also address the transparency of AI-generated content and encourage responsible use.