Generative AI for Dummies
Are you a non technical person looking to understand the basics of Generative Ai? You're in the right place!
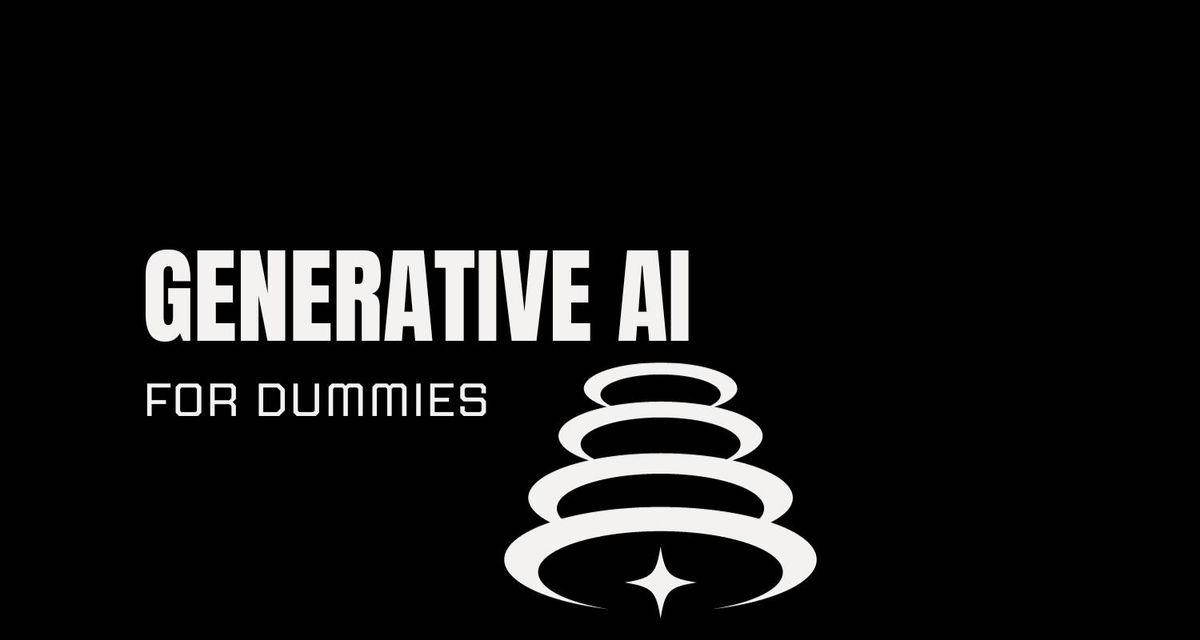
Are you a non technical person looking to understand the basics of Generative Ai? You're in the right place!
Diving into the realm of Artificial Intelligence (AI) can be intimidating, especially with the technical jargon that often accompanies it. However, fear not! This blog aims to simplify one of AI's intriguing aspects—Generative AI, for anyone curious about this digital frontier.
AI, from its inception in the 1950s to today, has been exhilarating. It began with creating machines that mimic human intelligence and has blossomed into various specialized branches. One such fascinating branch is Generative AI, which is not just about processing data but creating new, original content from it.
By the time you finish reading, you'll have a clearer picture of what Generative AI is, and why it's making waves in the business world.
Unfolding the concept of Generative AI is like opening a box of endless creative possibilities.
Let’s break it down into easily digestible tidbits.
What is Generative AI? (In Simple Terms)
Imagine having an invisible artist in your computer, one who could paint, compose music, or even write stories. This imaginative companion is what we call Generative AI. It's a type of artificial intelligence that's not just about crunching numbers or following commands. Instead, its main goal is to create—creating new content that is original realistic. Whether it's a picture, a tune, or a narrative, Generative AI dives into a pool of digital creativity to generate something fresh and new.
How Generative AI Differs from Other AI Systems?
If we compare traditional AI to a math genius who can solve complex problems, Generative AI is more like a creative artist who brings new ideas to life. While traditional AI analyzes and learns from existing data to make decisions or predictions, Generative AI uses its learnings to craft new data from scratch. It's like comparing a top-notch detective solving cases using clues (traditional AI) to a novelist creating a world of stories from imagination (Generative AI).
Generative AI Examples
Let's dive into a few real-world scenarios to see Generative AI in action:
- Digital Painting: Imagine a digital artist capable of creating diverse pieces of artwork. That's Generative AI for you! It can sketch, color, and produce visual art that is unique every time.
- Music Composition: Ever thought a machine could compose a melody? Generative AI does that. It can create tunes that would make you want to dance, capturing the essence of rhythm and melody.
- Creative Storytelling: From weaving whimsical tales to penning down poignant poetry, Generative AI can stretch its imaginative muscles quite far in the realm of writing.
- Designing Virtual Worlds: In the gaming universe, Generative AI helps in designing realistic, exciting game environments, making your virtual adventures more thrilling and immersive.
- Innovative Product Designs: It can also aid designers in coming up with innovative product designs, by generating a variety of design options and visualizations.
These examples underscore the ability of Generative AI to not just think, but imagine, making it a remarkable tool in various creative fields. The magic lies in its capacity to create, to bring something new to the table, every time it gets to work.
Generative AI vs AGI (Artificial General Intelligence)
Navigating through the landscape of Artificial Intelligence, we stumble upon a few different avenues.
Two such paths lead us to Generative AI and AGI (Artificial General Intelligence).
Let's venture into understanding how they relate and differ:
What is AGI in simple terms?
Artificial General Intelligence (AGI) is often seen as the holy grail of AI research. It refers to highly autonomous systems that have the ability to understand, learn, and apply its knowledge across a wide range of tasks just as a human would.
In simple words, AGI is like a multi-talented individual who can excel in different fields—be it art, science, sports or any other domain, much like an all-rounder who thrives in various scenarios.
Imagine the smartest and most creative person rolled up into a single machine.
The Key Differences Between Generative AI and AGI
While Generative AI is like a personal assistant, AGI is more of a universal genius.
Here’s how they differ:
- Scope of Application: Generative AI excels in creating new content within specified domains, whereas AGI has the ability to perform any intellectual task that a human being can do.
- Learning and Adaptation: Generative AI learns from specific datasets to generate new data creatively. On the other hand, AGI can learn, reason, and apply knowledge in different domains, adapting to new tasks with little to no human intervention.
- Level of Autonomy: While Generative AI requires a certain level of human guidance, AGI operates with a high degree of autonomy, being able to transfer knowledge from one domain to another effortlessly.
Why Generative AI is a Step Towards AGI but not the same
Generative AI showcases a glimpse of creativity, an essential human trait, by generating novel content and helping code. It's like a stepping stone that hints at the potential of machines possessing a level of understanding and creativity. However, it's still confined to specific domains and relies on human-prepared datasets.
On the flip side, AGI aims for a broader horizon, aspiring to match human intelligence across a wide spectrum of tasks and domains. It's about mastering the art of learning and reasoning in a way that can be applied universally, not just in creating new content.
While Generative AI opens a window to the expansive sky of machine creativity, AGI aims to soar across this sky, embodying a level of intelligence and versatility akin to a human. Hence, while Generative AI is a fascinating step forward, the journey towards achieving AGI is a marathon that continues to challenge and inspire the realm of artificial intelligence.
Generative AI vs LLM (Language Model)
Another term that comes up a lot when discussing AI is LLMs or Large Language Models.
Let's break down in simple terms what LLMs are and how they are different from generative AI
Understanding LLMs in Simple terms:
Large Language Models (LLMs) like GPT-3 (from OpenAI) are trained on extensive datasets encompassing text from books, websites, and other textual resources.
They are adept at processing and generating human-like text based on the input they receive. Their primary function revolves around understanding the context provided, predicting subsequent text, and formulating coherent and contextually relevant responses or narratives.
How Generative AI and LLM's work together:
Generative AI is a broader term that encompasses models capable of creating data that resembles data it was trained on.
LLMs fall under the umbrella of Generative AI as they too generate text based on the patterns learned during training.
While Generative AI can span across various forms of data generation such as images, text, or even sounds, LLM specifically pertains to text generation. Essentially, all LLMs are a subset of Generative AI, but not all Generative AI systems are LLMs.
Differences between Generative AI and Traditional Language Models:
Data Generation:
- Generative AI aims to create new data that mimics the distribution of the training data, whereas traditional language models primarily focus on understanding and processing text rather than generating new text.
Complexity:
- Generative AI, due to its creative aspect, often involves a higher degree of complexity compared to traditional language models which are generally tasked with simpler objectives like text classification, sentiment analysis, etc.
Learning Paradigm:
- Generative models learn the joint probability distribution of the data, while traditional language models learn the conditional probability distribution. This fundamental difference impacts how each model interacts with and processes data.
Training Data:
- The data required to train Generative AI models, especially LLMs, is significantly larger and diverse compared to that for traditional language models. This diverse data allows Generative AI and LLMs to have a broader understanding and ability to generate more creative and varied outputs.
Generative AI vs Machine Learning
So now that we have a simple understanding of Generative AI, AGI and LLMS, let's focus on how generative AI differs from machine learning and how they work together.
Definition of Machine Learning:
Machine Learning is a branch of AI that empowers computers to learn from data, improve with experience, and make predictions or take actions without being explicitly programmed. It thrives on algorithms that parse data, learn from it, and then apply the learned insights to make informed decisions.
Exploring How Generative AI Goes Beyond Traditional ML:
Data Generation:
- Unlike traditional ML which is more focused on making predictions, Generative AI excels in creating new data that shares statistical characteristics with the training data.
Creativity:
- Generative AI introduces an element of creativity, autonomously generating unique solutions, artwork, or text, while ML generally requires a defined dataset to work from.
The Difference in the Training Processes of Generative AI and ML:
Training Paradigm:
- Generative AI often employs adversarial training processes, like in Generative Adversarial Networks (GANs), while ML utilizes supervised, unsupervised, or semi-supervised learning methods.
Data Utilization:
- Generative AI requires a large amount of data to understand the underlying patterns for generation, whereas ML can often work with smaller datasets for prediction.
How Generative AI and ML Complement Each Other:
Enhanced Performance:
- ML can benefit from the data generated by Generative AI, especially in scenarios where data is scarce or expensive to acquire.
Complex Problem-Solving:
- Generative AI can provide creative solutions which, when combined with ML’s predictive analytics, can result in robust problem-solving frameworks.
Improved User Experiences:
- Integrating Generative AI’s creative generation with ML's analytical capabilities can lead to richer user experiences in applications like recommendation systems, virtual assistants, and many more.
Both Generative AI and Machine Learning hold immense potential, and understanding their functionalities and differences is important.
Their integration can lead to innovations that are beyond the sum of their parts, promising a future where AI’s capabilities are bound only by the extent of human imagination.
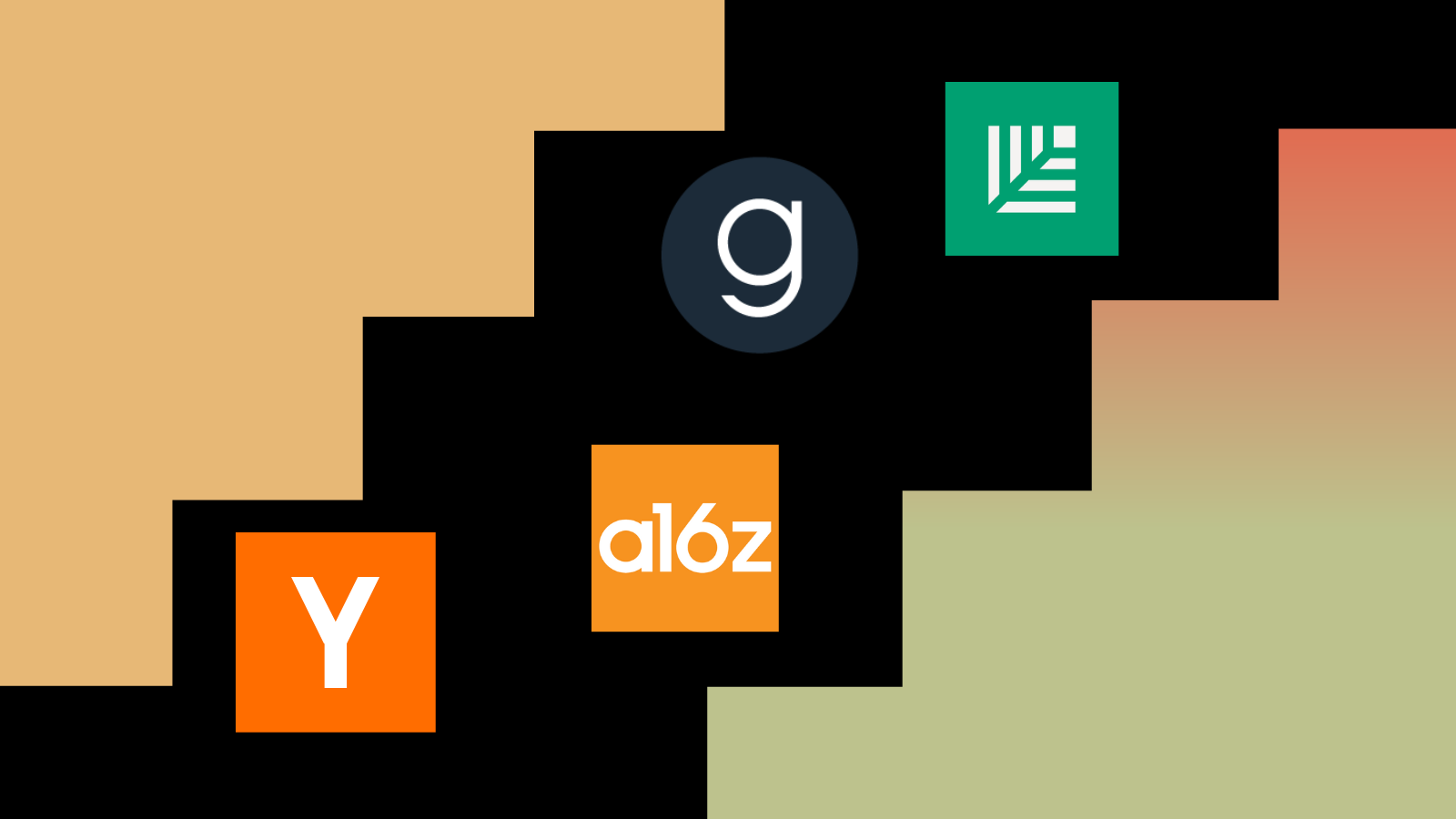
Business Applications of Generative AI
The strides in Generative AI technology are not merely a pursuit of academic or technological excellence, but they hold profound implications for the business domain.
Now that we have an understanding of AI and it's many components.
As businesses grapple with mass amounts of of data and the need for innovation, Generative AI emerges as a beacon of hope.
Let's explore how AI can impact businesses:
Product Development:
- Businesses are employing Generative AI for ideation and design in product development. By analyzing existing data, Generative AI can propose new product designs, potentially reducing the time and resources required in the initial phases of product creation.
Content Creation:
- Generative AI is becoming a valuable ally for content creators, aiding in generating textual, visual, and audio content, thus exponentially accelerating the content creation process while reducing costs.
Data Augmentation:
- In sectors where data is sparse, Generative AI can augment existing datasets, improving the performance of predictive models and analytics systems.
Customized Marketing:
- Personalized marketing campaigns can be designed with the help of Generative AI, by analyzing consumer behavior and generating content that resonates with target audiences.
AI Examples in Business:
From revolutionizing automotive designs to conjuring up trendy apparel and even hastening the pace of drug discovery, Generative AI is proving to be a game changer.
Here's a glimpse of how different sectors are harnessing the creative prowess of Generative AI to drive a new wave of advancements.
Automotive Industry:
- BMW utilized Generative Design algorithms to optimize the design of their i8 Roadster, shedding weight while maintaining structural integrity.
Fashion and Retail:
- Stitch Fix, an online personal styling service, employs Generative AI to create new apparel designs based on customer preferences and historical data.
Pharmaceuticals:
- Atomwise employs Generative AI for drug discovery, aiding in the generation of compound structures that could potentially lead to new therapeutic drugs.
Future Trends in Generative AI for Business:
Robust Prototyping:
- The potential of Generative AI in creating more accurate prototypes swiftly can lead to faster product launches and market adaptation.
Virtual Reality (VR) and Augmented Reality (AR):
- As VR and AR technologies mature, Generative AI could play a pivotal role in creating realistic, interactive, and dynamic virtual environments for a multitude of business applications.
Real-Time Decision Making:
- Integrating Generative AI with real-time analytics could enable businesses to make informed decisions promptly, reacting to market changes with unprecedented agility.
Ethical and Regulatory Evolution:
- As Generative AI continues to grow, ethical considerations and regulatory frameworks will evolve to ensure responsible and fair utilization of this powerful technology.
Conclusion
Generative AI is a rapidly evolving field that offers a wide range of applications and opportunities. From creating new art and music to designing new products and treatments, generative AI has the potential to transform the way we live and work.
If you're interested in learning more about AI and companies receiving funding
Subscribe to our weekly email newsletter that posts where the money is flowing in AI